Jishu Mehdi Ph.D. Dissertation Defense - Reliable, Secure and Safe Deep Learning
- Kent
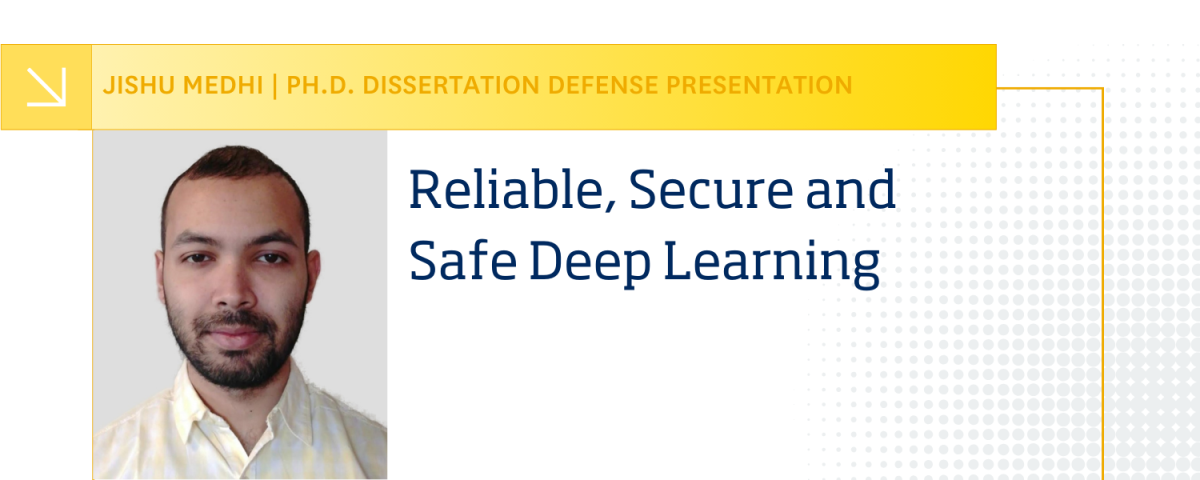
Deep learning has propelled the advancement of artificial intelligence in recent times by providing powerful tools for data analysis, pattern recognition, and decision-making. Its ability to learn intricate patterns and representations from data has led to remarkable breakthroughs across the domain of image recognition, natural language processing, healthcare, and robotics. However, the reliance on complex neural networks brings forth challenges related to their reliability, security, and safety. For example, the lack of high-quality data labels may introduce errors and biases into the training process and lead the model to poor performance and unreliable predictions, but labeling large datasets for deep neural networks is quite expensive, time-consuming, and labor-intensive. In addition, adversarial attacks on deep neural network training can not only destabilize the learning process but also compromise the privacy of training data and disregard the safety of task execution. In this work, we discuss the inherent vulnerabilities of deep learning systems and explore strategies to enhance their robustness, reliability, privacy, security, and safety. We delve into issues such as adversarial attacks, data quality, model privacy, and multiagent consensus problem, proposing novel solutions and implementing best practices to mitigate these challenges.
This dissertation addresses some of these concerns by specifically focusing on four key research problems: (1) improve the reliability of deep learning in applications that are impacted by the issues of data sparsity and object sensitivity using multi-task learning, (2) address data privacy and security concerns when outsourcing deep learning to an untrusted cloud server (3) mitigate the vulnerabilities in multiagent reinforcement learning (MARL) resulting from a Byzantine attack, and (4) propose a novel intrusion detection system to address the risks posed by cyberattacks on unmanned aerial vehicles (UAV). By addressing these concerns, we aim to pave the way for the development of more dependable, privacy aware and secure deep learning systems.